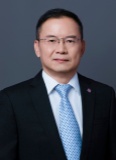
|
Prof. Qing LiThe Hong Kong Polytechnic University, Hong Kong, China IEEE Fellow
Qing Li is a Chair Professor and Head of the Department of Computing, the Hong Kong Polytechnic University. He received his B.Eng. from Hunan University (Changsha), and M.Sc. and Ph.D. degrees from the University of Southern California (Los Angeles), all in computer science. His research interests include multi-modal data management, conceptual data modeling, social media, Web services, and e-learning systems. He has authored/co-authored over 500 publications in these areas,with over 45,000 citations and H-index of 87 (source: Google Scholars). He is actively involved in the research community and has served as Editor-in-Chief of Computer & Education: X Realitty (CEXR) by Elsevier, associate editor of IEEE Transactions on Artificial Intelligence (TAI), IEEE Transactions on Cognitive and Developmental Systems (TCDS), IEEE Transactions on Knowledge and Data Engineering (TKDE), ACM Transactions on Internet Technology (TOIT), Data Science and Engineering (DSE), and World Wide Web (WWW) Journal, in addition to being a Conference and Program Chair/Co-Chair of numerous major international conferences. He also sits/sat in the Steering Committees of DASFAA, ER, ACM RecSys, IEEE U-MEDIA, and ICWL. Prof. Li is a Fellow of IEEE, AAIA, and IET/IEE. Title: A Multi-level Querying Method for an Indoor Robot Smart Space Abstract: Smart Space denotes dynamic, adaptive environments enhanced with robotics and AI technologies. Examples include smart homes/offices/cafes. By leveraging and integrating Computer Vision, Natural Language Processing, AIoT, Data Mining, Recommender Systems, and Sympathetic Computing, Smart Space can help improve efficiency, personalization, and user satisfactions with seamless interactions. In this talk, we introduce PolyRAG, a multi-level knowledge QA framework supporting multi-level querying for an indoor robot application system. Building on top of a naive RAG layer, we construct a knowledge pyramid by adding a knowledge graph layer and an ontology schema, so as to obtain a good balance of recall and precision when applied to a specific domain such as coffee robot interactions. An experimental coffee robot prototype is implemented, and preliminary empirical studies are conducted to show the effectiveness of our PolyRAG supporting top-down querying from ontology to KG to RAG.
|